Trayned Pioneer launch!
Welcome to Trayned Pioneer, a comprehensive and easy to use tool that presents data on performance in all GP practices in England in a simple and intuitive way. Trayned Pioneer has been developed by Trayned Insight, an independent organisation that has applied experience gained over 25 years in the analysis of information for the financial services sector, to open source health service data. We have used a novel statistical methodology to analyse and present data in a more intelligible and valid way so that it can be viewed and and interrogated at the same time by a user with minimal training or by a skilled data analyst. At the heart of our approach is the notion that if we can identify variance in commonly used measures of performance we can direct practitioners and commissioners to the most important areas of variation. We do not attempt to explain cause, but present what we think is significant variation in order to highlight the true opportunities for improvement at a practice level. Trayned Pioneer uses a statistical approach that allows comparison of practice level performance across the whole of the country.
"Experience gained over 25 years"
This approach is based on the premise that when comparing organisations that differ widely in many parameters it is necessary to identify which parameters are most closely shared, and which factors affect outcomes. Our database is built around a large number of openly available datasets, including national census data, the multiple Index of deprivation, and a host of NHS performance, prevalence and prescribing data. We have used common NHS performance indicators as the initial question set. Trayned Insight has pioneered an approach that we have called the "Nearest 99”. This requires segmentation of all primary care practices, surgeries and pharmacies across England on the basis of shared characteristics so that we can make valid comparisons of performance based on those shared characteristics. In this way we provide a more statistically valid measure of variation in performance within any given practice, and for any given parameter. The data can be easily aggregated at the CCG, STP or NHS(E) level. The selection of the “Nearest 99” varies for each performance indicator, thus taking account of the importance of weighting different factors in assessment of different performance indicators. This approach exposes variations in performance that are traditionally masked within current approaches.
Trayned Pioneer thus overcomes one of the most common objections to the application of numerical statistics to compare individual units in any field. The typical response when variation is highlighted is to say, “ah, but we are different to all those others because ….”. These objectors seek to dodge the perceived criticism and evade any need to change. To be fair, this common sense objection has some justification; most approaches to statistical comparison start by trying to get an assessment of everyone first – the “population”, and then seeing how any particular individual compares to this population norm.
"Trayned Insight has pioneered an approach that we have called the "Nearest 99”"
To give an example, if we know that the average height that people can jump is approximately 40cm and two people jump 30cm and 50cm respectively, we might immediately come to a conclusion that the first is less fit than the second. However, the first might be a 70 year old woman, and the second a 25 year old man. Compared with other 70 year old women, 30 cm is an excellent achievement, whilst compared to other 25 year old men, 50 cm is quite poor. This becomes even more critical when seeking to generate personalised medicine, where ideally the responses to a treatment are assessed with reference to a pool of patients who are as similar to the presenting individual as possible. More relevantly in our own circumstances, we want to find a pool of GP practices that are most similar to each particular GP practice being assessed.
Our approach to segmentation and the production of a frame of reference to compare any particular GP practice, dental surgery or pharmacy, is to build up from this individualized perspective. We believe that this is the real opportunity that access to large volumes of data provides. Rather than assuming that large volumes of data will be able to provide ever closer accuracy, with the grave risk that we are generating over confidence in any results, we use the data to identify micro-samples that treat each individual unit as its own frame of reference.
In this way we have developed an application that balances the competing demands for relevance and robustness for any indicator and NHS unit. What we mean by relevance is finding the other practices that share as many detailed characteristics as possible with the target practice. We want to find the most similar units within the comprehensive datasets available. By robustness, we mean that the conclusions should as far as possible persist and not be an artefact of the individual units selected for the micro-sample. We also want our information to be as easily interpreted by a practitioner, as by a data expert. To that end, to identify the most relevant practices to use in a micro-sample we consider all the available data that covers the characteristics of the practice list, its population and organisational structure. At the level of Lower Super Output Areas (LSOAs), defined by the office of national statistics, we can apply weightings to characteristics of the practice list. Characteristics include several domains: (1) deprivation, (2) age profiles, (3) gender make up, (4) the proportion of patients within care-homes, (5) census variables that cover ethnicity, (6) household make up, (7) country of birth and (8) religion. In addition, we also utilise the prevalence rates that are published for practices within the Quality Outcomes Framework and additional practice structure variables, like list size, patient satisfaction scores and number of GPs. Within this extensive list we rank each variable, with 1 being the lowest value, up to the number of practices being compared, with tied values being assigned the mean rank. For each practice we then find the 99 other practices that have the most similar ranks, based on a measure of similarity that conducts a weighted addition of the absolute difference between the ranks on each variable. The weights are specific to each indicator under consideration as this reflects the overall importance of particular variables to each indicator.
"We have developed an application that balances the competing demands for relevance and robustness"
Robustness is then balanced with the selection of similar practices by the selection of a fixed micro-sample size for each practice, hence the “Nearest 99. This constrains the influence of any individual practice in the comparison, both by making sure there are always another 98 contributions, but also by utilising the ranks rather than measured values.
Within this micro-sample it is straightforward to identify what rank the particular practice of interest has compared to other practices within this sample of similar practices. The ranking of any given practice references its position within its nearest 99 segment. With a lower rank always being representative of poorer performance.
In order to reduce clutter within some of the charts we further simplify the output at times by referencing the deciles for each variable, e.g. the lowest decile, 1, contains ranks from 1 to 10, highest decile 10, contains ranks 91 to 100.
To return to the original objection, we have built this approach so that when we highlight variation in performance the user can be confident that it is significant. The user must then consider the cause of that variation, perhaps by reference to different ways of working in any chosen area of practice. The tool will also quantify the size, and often the cost of the variation, and can track changes and benefits in line with periodic release of new data.
Meet the team
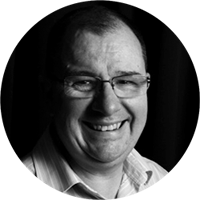
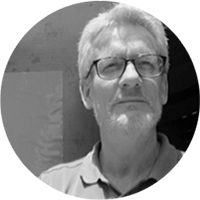
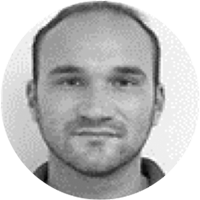
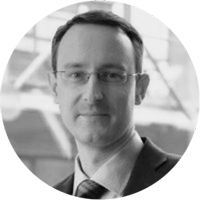
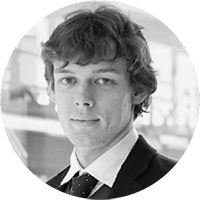
FROM OUR BLOG
Latest Insights
Learn more about
Trayned Pioneer
+44 (0) 20 86 00 23 00